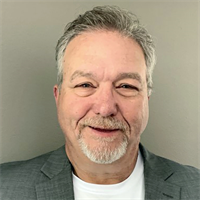
Integrating Advanced Data Analytics into Metal Forming Operations
January 9, 2024Comments
The metal forming industry faces complex challenges, ranging from increasing material costs to stringent quality standards. Utilizing data analytics promises to enhance operational efficiency and equips businesses with the tools they need to adapt swiftly to market changes and customer needs.
Precision in metal forming represents much more than just a way to maintain quality. It's also a critical factor in ensuring cost-effectiveness and resource optimization. Data analytics plays a vital role here, offering insights into process parameters and enabling manufacturers to achieve the highest levels of precision.
With the following data-driven processes, companies can significantly enhance their operational efficiency:
- Real-time monitoring through data analytics transforms how companies manage their metal forming operations. Data analytics allows managers to immediately identify and correct process deviations, ensuring optimal performance. This proactive approach to process control boosts efficiency and minimizes the risk of defects and rework, leading to reliable and consistent production outcomes.
- Predictive analytics serves as a powerful tool in extending machine life and proactively addressing maintenance needs. It allows companies to analyze patterns and predict tool wear in order to proactively schedule maintenance activities and avoid unexpected downtime. This predictive approach enhances tool lifespan while enabling smooth and uninterrupted operations.
Reduced Reject Rates, Refined Process Accuracy
For a practical example of data analytics in action, consider its application in reducing reject rates. Through detailed data analysis, manufacturers can fine-tune their processes and make precise adjustments to address quality issues. The result: reduced reject rates and enhanced efficiency and product quality.
Closed-loop control systems, guided by data analytics, continually adjust operations based on real-time data, ensuring that production stays within specified tolerances. These systems improve process reliability and consistency, essential for maintaining quality standards.
Too, machine-learning algorithms are revolutionizing process-parameter optimization by analyzing vast amounts of production data, which helps identify patterns and optimize parameters for peak performance. This adaptive approach results in processes with improved accuracy and efficiency, reducing waste and increasing output quality.
One tangible outcome of employing data analytics is the achievement of micron-level tolerances. Leveraging data for precise control of machinery and processes enables metal formers to produce components with exceptional accuracy.