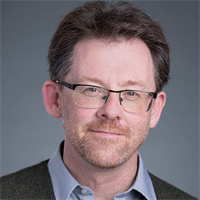
Machine Learning: A Game Changer for AM Quality Assurance
November 11, 2020Comments
Each of us has a preconception of what the term “machine learning” means. According to ExpertSystems.com, “Machine learning (ML) is an application of artificial intelligence (AI) that provides systems the ability to automatically learn and improve from experience without being explicitly programmed.”
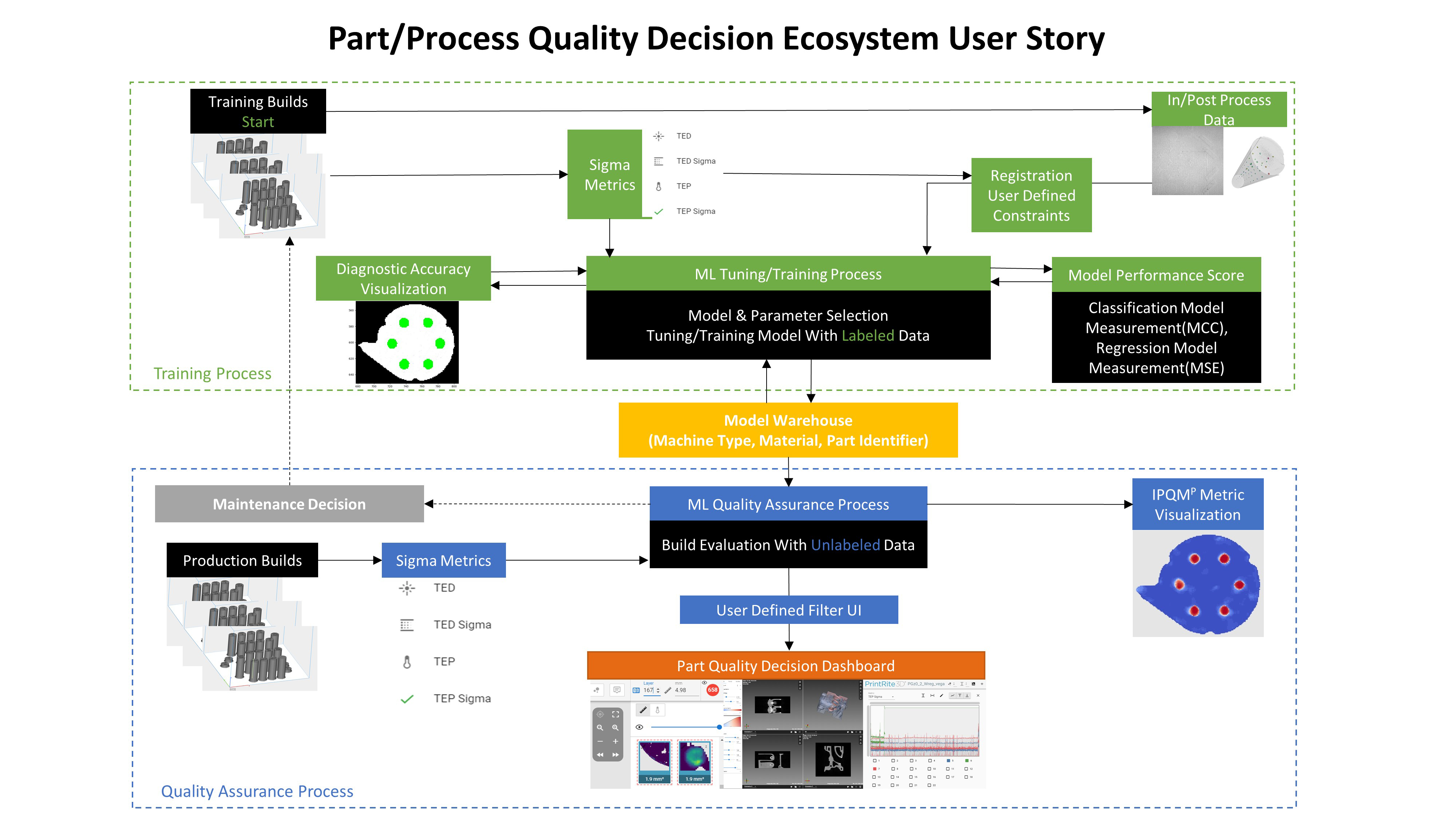
Machine learning implies that systems can learn from data, identify patterns and make decisions with minimal human intervention. ML focuses on the development of computer programs that can access data and then allow machines to use that data and learn for themselves.
Machine learning in any environment, including metal AM, depends on gathering quality data and then using the right system to validate that data. Like most other processes, the old expression “garbage in garbage out” applies.
Does ML function in the real-world of AM? The answer is a resounding yes. As noted in a recent 3DPrinting.com report, “Porosity and other defects are a problem where it comes to parts printed with metal powder-bed fusion processes. One team of researchers from the U.S. Department of Energy’s Argonne National Laboratory and Texas A&M University has published its findings that may play a part in reducing these subsurface defects. The team has figured out a novel way of predicting the formation of subsurface porosity as well as measuring the temperature of a region at the very moment of printing of Ti-6Al-4V powder.
“Specifically, the researchers were interested in predicting the formation of keyhole pores,” the online article continues, “which are formed when a surplus of energy is concentrated in the metal melt pool for too long. These pores can act as stress concentrators in the final part’s structure, leading to part unpredictability under load, and potential failures. By use of a top-down high-speed thermal camera in the build area of the laser fusion printer they are able to log the relative temperature at a certain time during printing, and correlate that with additional scans from synchrotron x-ray imaging taken at the exact same moment. This helps to build a picture of how the thermal history of the fused part affects the microstructure of the printed metal.”